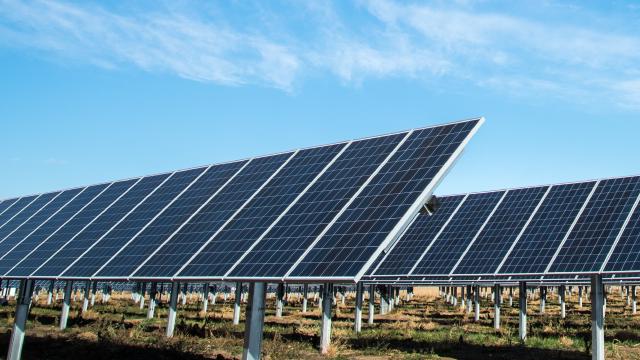
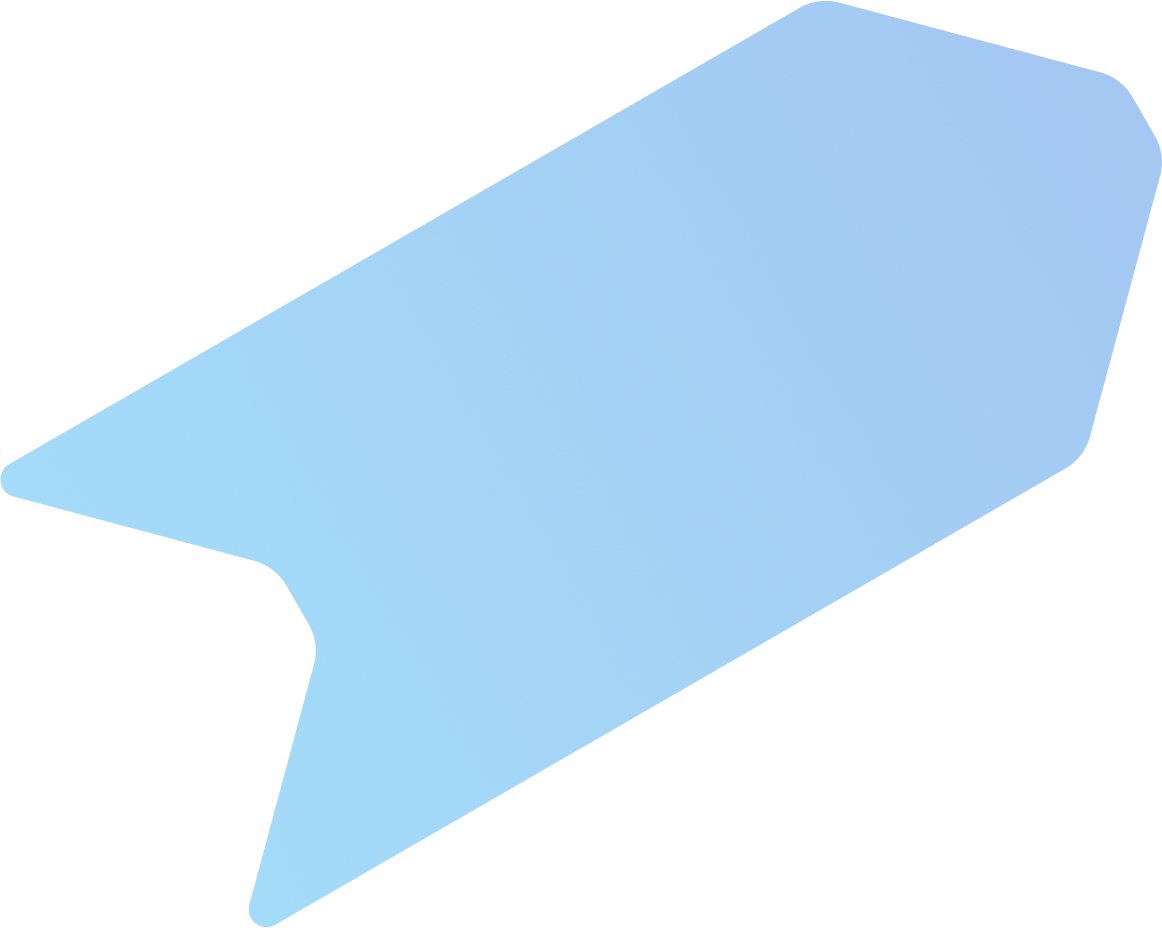
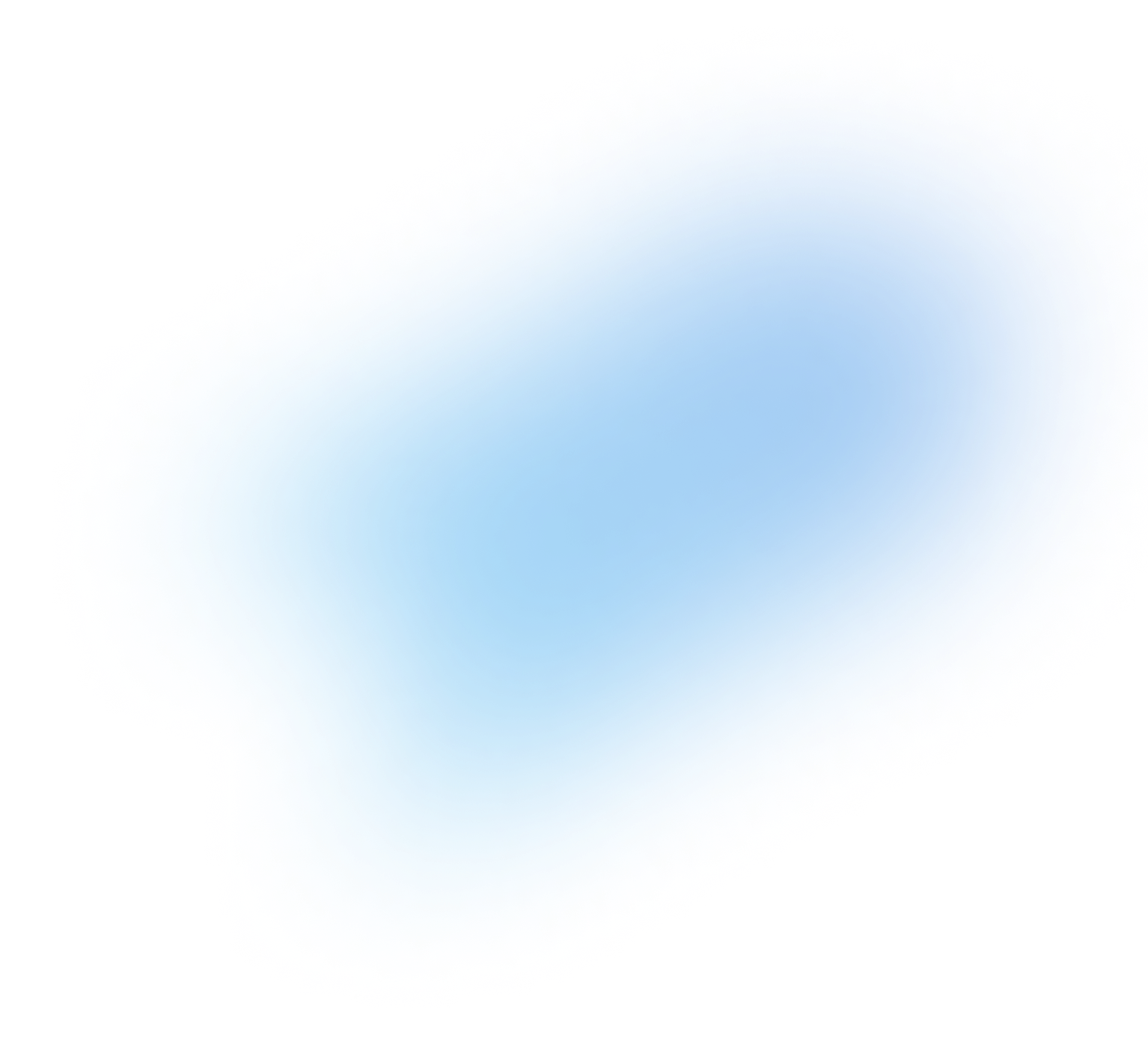
CASE STUDY
Demand Forecasting and Inventory Allocation Optimization Project for Snow Tires
Optimizing demand forecasting and inventory allocation for snow tires using AI, and simultaneously reducing operating costs and the loss of opportunity due to stock-out
Project Year: 2022
Client: Bridgestone Corporation
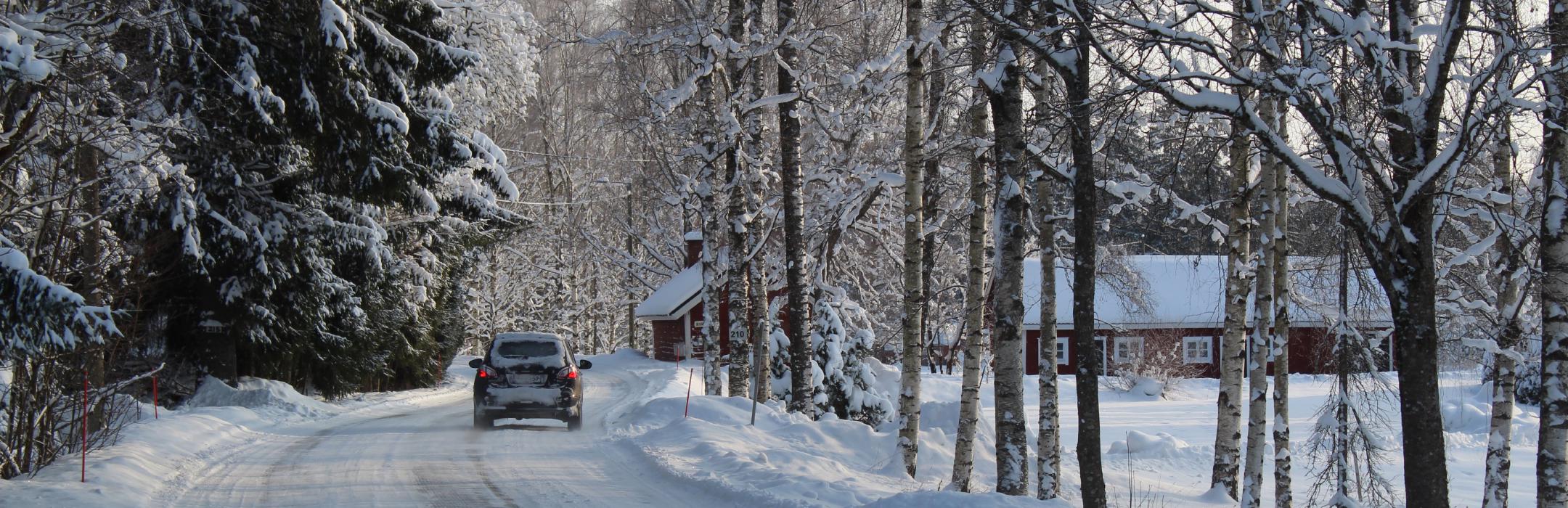
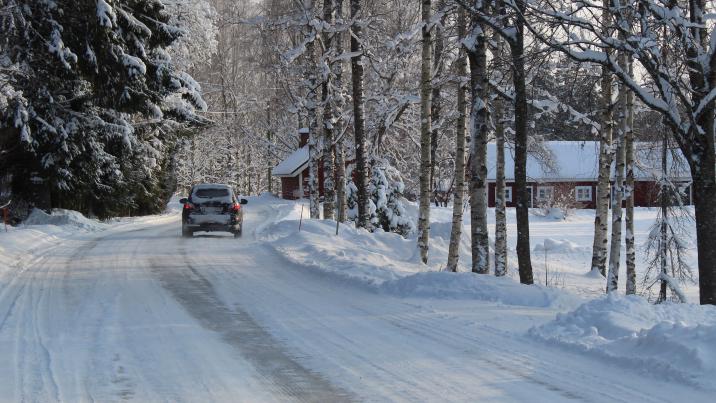
Issue
Snow tires, including studless tires, are optimized for driving on snow-covered roads. It is not easy to estimate the sales volume for a particular year. The sales volume is mainly affected by the following three factors.
Weather condition Compared to summer tires, demand for snow tires is affected to a greater extent by temperature and weather such as snowfall. In snowy areas, demand fluctuates depending on the timing of snowfall, while in non-snowy areas, demand suddenly increases when there is unexpected snowfall. Although the sales volume estimate is based on the average weather conditions each year, there are often deviations.
Availability of other companies’ products Dealers sell tires from other companies. When tires from one manufacturer are out of stock, tires from another manufacturer are often purchased. The availability of other companies’ products also affects the sales volume.
Sales status of new cars Tire sizes vary by the types of cars. Therefore, the tire size in demand is determined by the sales trend of new cars.
As explained above, the sales volume of snow tires is affected by multiple factors. In the past, persons in charge of sales made forecasts based on their experience. However, every time the forecasts happened to be wrong, they had to deal with inventory being out of stock or overstocks. This resulted in additional operating costs, such as extra work to fix the problems, and losses due to lost sales opportunities.
An Approach to Solving the Issue
We developed the following two AI models to simultaneously reduce operating costs and the loss of opportunity due to out-of-stock tire inventory.
- Demand forecasting model that forecasts the sales volume of tires
- Inventory allocation optimization model that allocates inventory to appropriate warehouses according to the demand forecast result
Demand forecasting model
- We developed a demand forecasting model by adding weather and car sales data as features to tire sales data and using machine learning that can adapt to weather changes.
- In some areas with insufficient snowfall data, there was a limitation on machine learning. We also used statistical processing for forecasting to develop a hybrid model of statistics and machine learning.
Inventory allocation optimization model
- We optimized inventory allocation based on the results of the demand forecast.
- We developed an optimization model that calculated how many products should be allocated to which areas and at what time and then developed a shipment plan to achieve that allocation.
Data used
- Operational data, such as orders received, shipments, inventory, and out-of-stock tire inventory
- External data, such as snowfall and new car sales
Effect
- We realized flexible inventory allocation where products are intensively sent from factories to areas that need the products and inventory is moved from north to south, depending on when sales peak.
- We applied real data to the demand forecasting model and found that the accuracy of the forecast for key products, which account for 90% of total sales, was over 80%.
- We succeeded in reducing the operating costs and eliminating the loss of opportunity due to out-of-stock tire inventory.
- In order to expand the inventory allocation model, which is currently used only in some regions, to the whole country, we are improving the model and establishing an operational mechanism.
Project Members
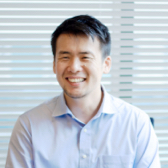
Keiichiro Kitada
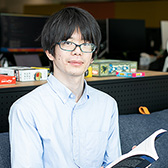
Ryota Matoba
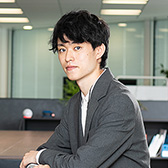
Ryoto Suzuki