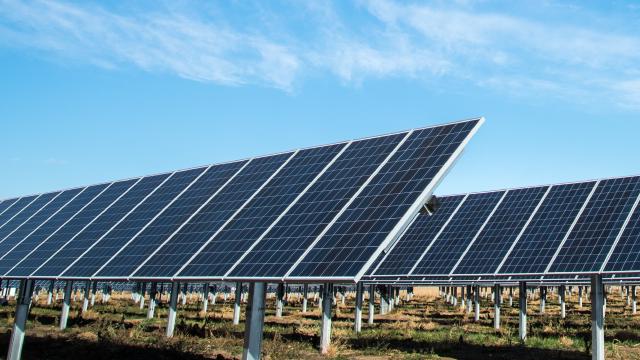
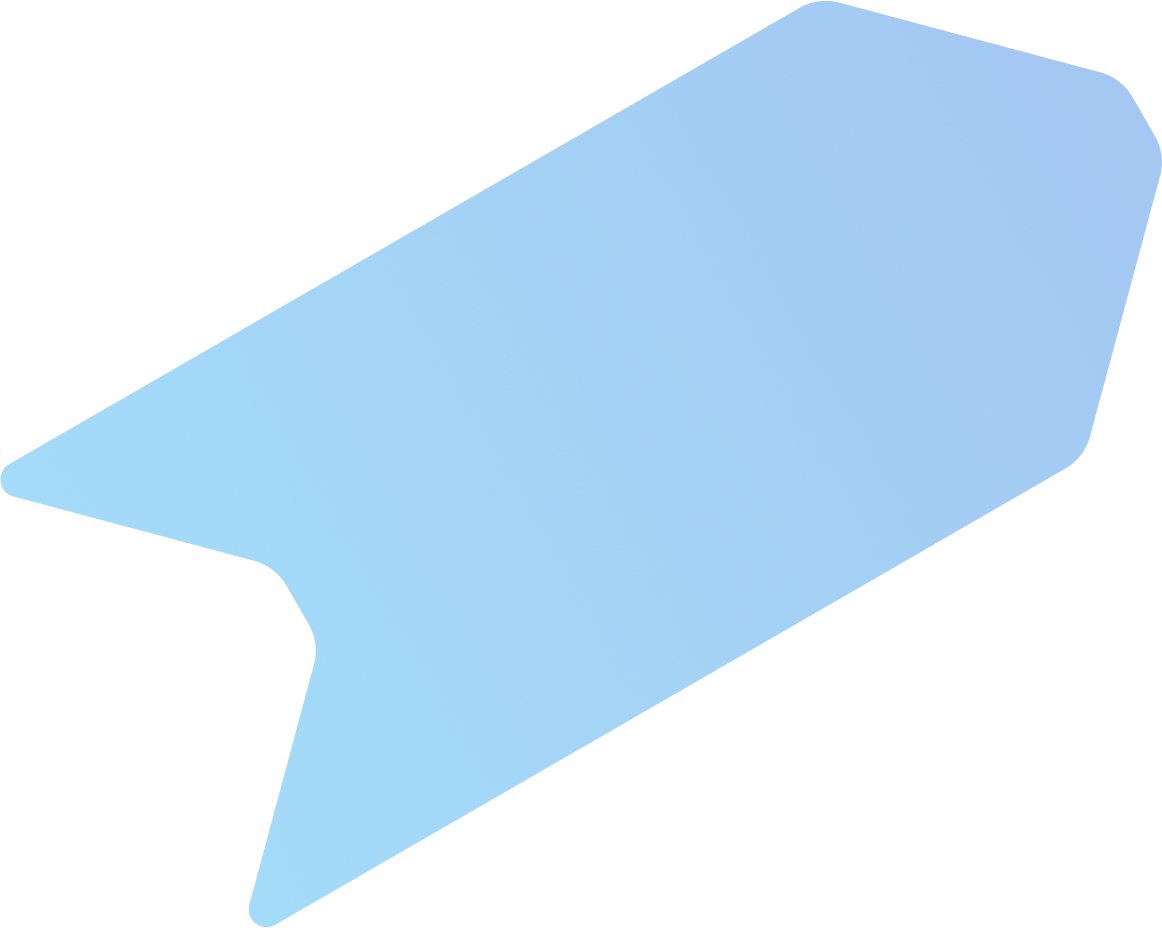
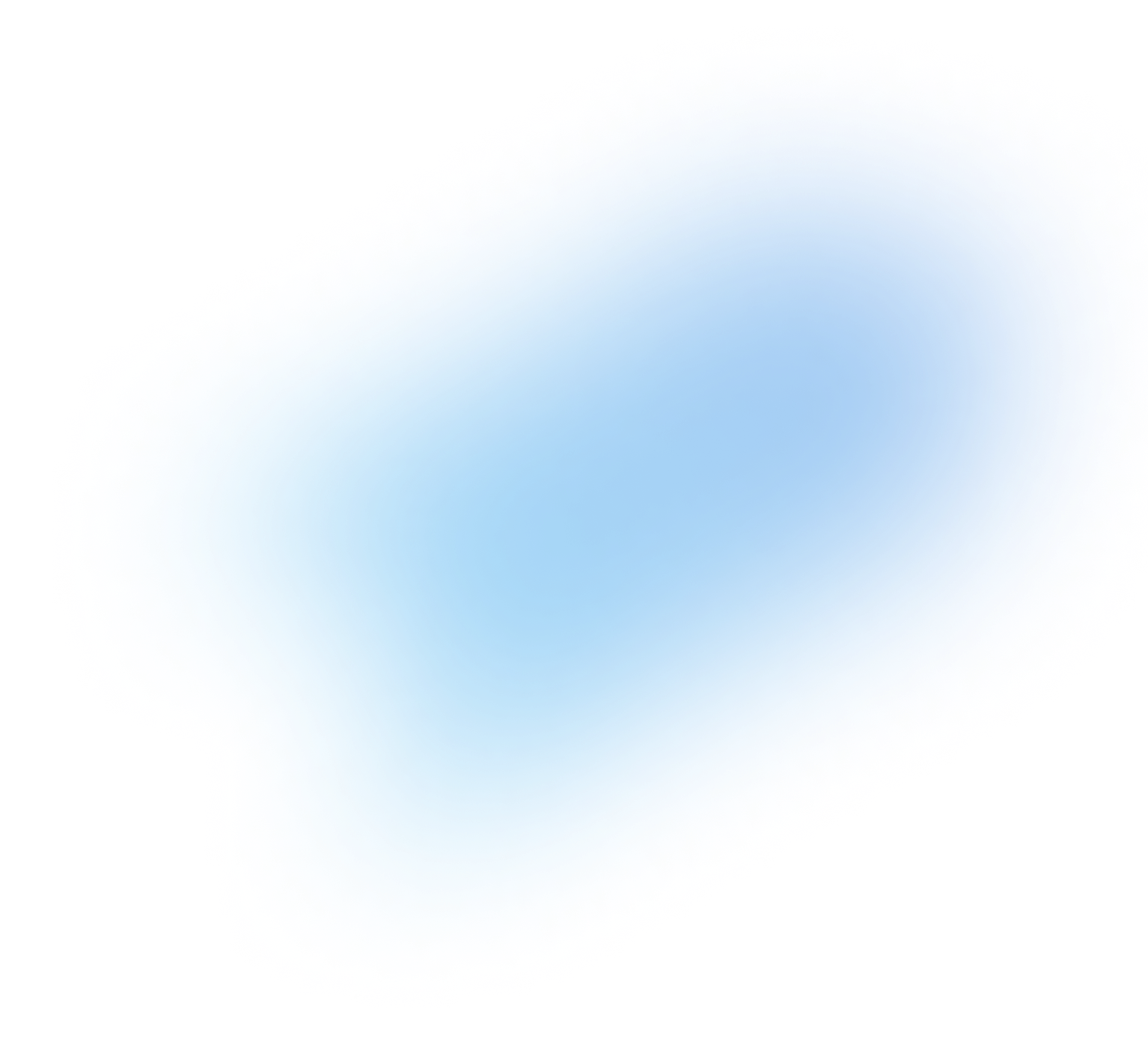
CASE STUDY
Project to Predict the Amount of Electricity Generated by Solar Panels
An Initiative to Minimize the Error of the Predicted Amount of Electricity Generation in order to Reduce the Costs of Penalties
Project Year: 2022
Client: Mitsubishi Corporation
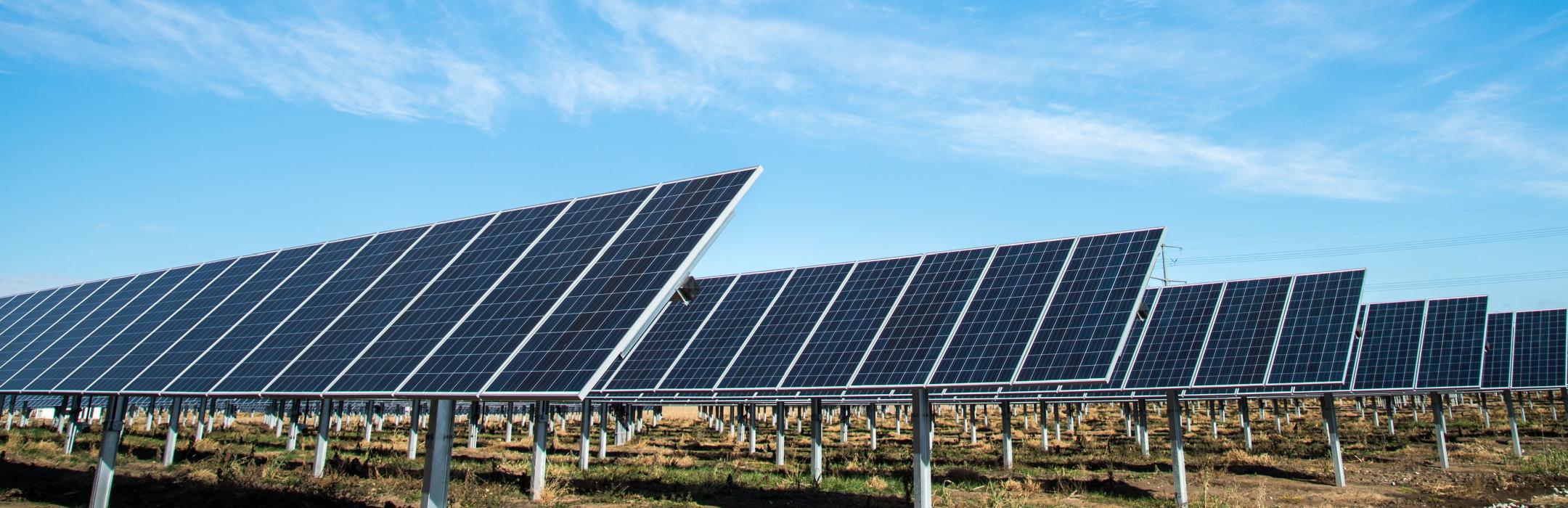
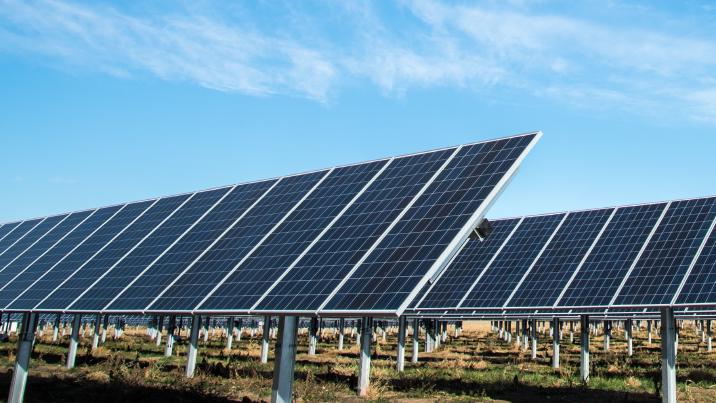
The Environment surrounding the Electric Power Industry
Climate change is one of the most pressing issues that directly affects industries and people’s lives around the world.
To tackle this issue, the government set a target in 2020 to achieve net zero greenhouse gas emissions by 2050.
To contribute to the achievement of this target, Mitsubishi Corporation also set the goal of creating new value through both energy transformation (EX) and digital transformation (DX).
Issue
One of the EX initiatives is to expand the renewable energy business. Supply and demand must be matched in the electricity business. However, this matching is more difficult than conventional electricity generation methods because the production of renewable energy depends on natural conditions. In addition, a system called the Feed-in Premium (FIP) program was introduced in 2022. Under the program, power producers must submit their planned amount of electricity generation in advance. Any deviation from the planned amount may result in the payment of imbalance penalties. With the launch of this program, accurately predicting of the amount of electricity to be generated has become key to reducing costs. Under these circumstances, a more accurate method for predicting the amount of electricity to be generated from solar power has been sought.
An Approach to Solving the Issue
In the early stage, before we had accumulated enough data, we used engineering models that did not require learning and could be built based on the mathematical and physical rules. Then, we chose to move to a machine learning model when we had accumulated a certain amount of data.
As engineering models, we developed models in three stages: a model that predicts the effective radiation based on the data of the sun’s direction and altitude, a model that predicts the temperature of the panels, and a model that predicts the amount of electricity to be generated based on the outputs of these two models. In the machine learning model, we validated approximately 50 experiments, including feature engineering and hyperparameter tuning, and adopted the one that gave the best result.
Data used
- Meteorological data including radiation, temperature, and cloud cover
- Data on the amount of electricity generated
Effect
We were able to keep the mean error (MAE) of the monthly prediction at a given location within 8% in both the engineering and machine learning models.
We were able to keep the MAE of the date/time-based prediction at all four target locations within 0.1% with a probability of more than 75% in the machine learning model.
By minimizing the error of the predicted amount of electricity generation with a more accurate model, the cost of imbalance penalties is expected to be reduced.
We are examining the possibility of developing SaaS or applying it to the prediction of the generation of other renewable energies, such as wind power, using the knowledge obtained from this project.
Project Members
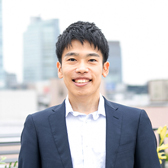
Hiroaki Tanaka